Enhanching Gravitational Wave Science with Machine Learning
Elena Cuoco, Jade Powell, Marco Cavaglià, Kendall Ackley, Michał Bejger, Dr. Chayan Chatterjee, Michael Coughlin, Scott Coughlin, Paul Easter, Reed Essick, Hunter Gabbard, Timothy Gebhard, Shaon Ghosh, Leïla Haegel, Alberto Iess, David Keitel, Zsuzsa Márka, Szabolcs Márka, Filip Morawski, Tri Nguyen, Rich Ormiston, Michael Pürrer, Massimiliano Razzano, Kai Staats, Gabriele Vajente, Daniel Williams
December, 2020
Abstract
Machine learning has emerged as a popular and powerful approach for solving problems in astrophysics. We review applications of machine learning techniques for the analysis of ground-based gravitational-wave (GW) detector data. Examples include techniques for improving the sensitivity of Advanced Laser Interferometer GW Observatory and Advanced Virgo GW searches, methods for fast measurements of the astrophysical parameters of GW sources, and algorithms for reduction and characterization of non-astrophysical detector noise. These applications demonstrate how machine learning techniques may be harnessed to enhance the science that is possible with current and future GW detectors.
Publication
In Machine Learning Science and Technology
Click the Cite button above to demo the feature to enable visitors to import publication metadata into their reference management software.
Create your slides in Markdown - click the Slides button to check out the example.
Supplementary notes can be added here, including code, math, and images.
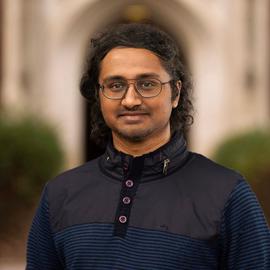
AI for New Messengers Postdoctoral Fellow
Dr. Chayan Chatterjee is the A.I. for New Messengers Postdoctoral Fellow at Vanderbilt University. His research focuses on application of machine learning to study gravitational waves - tiny ripples in space-time created by colliding black holes and neutron stars.